CHAPTER 11: REASONING & DECISION-MAKING
Let us consider the following scene of Knut’s life: It is a rainy summer afternoon in Germany and Knut and his wife are tired of watching the black crows in their garden. They decide to escape from the dreary weather and take a vacation to Spain, as Knut and his wife have never been there before. They will leave the next day, and he is packing his bag. He packs the crucial things first: underwear, socks, pajamas, and a toiletry bag with a toothbrush, shampoo, soap, sun tan lotion, and bug spray. Knut cannot find the bug spray, and his wife volunteers to go buy a new bottle. He advises her to take an umbrella for the walk to the pharmacy as it is raining outside, and then he turns back to packing. But what did he already pack into his bag? Immediately, he remembers and continues, putting together outfits and packing his clothing. Since it is summer, Knut packs mostly shorts and t-shirts. After half an hour, he is finally convinced that he has done everything necessary for a nice vacation.
With this story of Knut’s vacation preparation, we will explain the basic principles of reasoning and decision making. We will demonstrate how much cognitive work is necessary for even this fragment of everyday life.
In reasoning, available information is taken into account in the form of premises. A conclusion is reached on the basis of these premises through a process of inference. The content of the conclusion goes beyond either one of the premises. To demonstrate, consider the following consideration Knut makes before planning his vacation:
Premise 1: In all countries in southern Europe it is warm during summer.
Premise 2: Spain is a country in southern Europe.
Conclusion: Therefore, in Spain it is warm during summer.
The conclusion in this example follows directly from the premises, but it includes information that is not explicitly stated in the premises. This is a typical feature of a process of reasoning. We will discuss the two major kinds of reasoning, inductive reasoning and deductive reasoning, which logically complement of one another.
DEDUCTIVE REASONING
Deductive reasoning is concerned with syllogisms in which the conclusion follows logically from the premises. The following example about Knut makes this process clear:
Premise 1: Knut knows: If it is warm, one needs shorts and t-shirts.
Premise 2: He also knows that it is warm in Spain during summer.
Conclusion: Therefore, Knut reasons that he needs shorts and t-shirts in Spain.
In this example it is obvious that the premises are about rather general information, and the resulting conclusion is about a more special case which can be inferred from the two premises. We will now differentiate between the two major kinds of syllogisms: categorical and conditional syllogisms.
CATEGORICAL SYLLOGISMS
In categorical syllogisms, the statements of the premises typically begin with “all”, “none” or “some” and the conclusion starts with “therefore,” “thus,” or “hence.” These kinds of syllogisms describe a relationship between two categories. In the example given above in the introduction of deductive reasoning these categories are Spain and the need for shorts and T- Shirts. Two different approaches serve the study of categorical syllogisms: the normative approach and the descriptive approach.
THE NORMATIVE APPROAC H
The normative approach to categorical syllogisms is based on logic and deals with the problem of categorizing conclusions as either valid or invalid. “Valid” means that the conclusion follows logically from the premises whereas “invalid” means the contrary. Two basic principles and a method called Euler Circles have been developed to help make validity judgments. The first principle was created by Aristotle, and states “If the two premises are true, the conclusion of a valid syllogism must be true” (cp. Goldstein, 2005). The second principle states “The validity of a syllogism is determined only by its form, not its content.” These two principles explain why the following syllogism is (surprisingly) valid:
All flowers are animals. All animals can jump.
Therefore, all flowers can jump.
Figure 1. Euler Circles
Even though it is quite obvious that the first premise is not true and further that the conclusion is not true, the whole syllogism is still valid. That is, when you apply formal logic to the syllogism in the example, the conclusion is valid.
It is possible to display a syllogism formally with symbols or letters and explain its relationship graphically with the help of diagrams. One way to demonstrate a premise graphically is to use Euler circles (pronounced “oyler”). Starting with a circle to represent the first premise and adding one or more circles for the second one (Figure 1), one can compare the constructed diagrams with the conclusion. The displayed syllogism in Figure 1 is obviously valid. The conclusion shows that everything that can jump contains animals which again contains flowers. This aligns with the two premises which point out that flowers are animals and thus are able to jump. Euler circles help represent such logic.
THE DESCRIPTIVE APPROAC H
The descriptive approach is concerned with estimating people’s ability to judge the validity of syllogisms and explaining errors people make. This psychological approach uses two methods in order to study people’s performance:
Method of evaluation: People are given two premises and a conclusion. Their task is to judge whether the syllogism is valid.
Method of production: Participants are given two premises. Their task is to develop a logically valid conclusion.
In addition to the form of a syllogism, the content can influence a person’s decision and cause the person to neglect logical thinking. The belief bias states that people tend to judge syllogisms with believable conclusions as valid, while they tend to judge syllogisms with unbelievable conclusions as invalid. Given a conclusion as like “Some bananas are pink”, hardly any participants would judge the syllogism as valid, even though it might be logically valid according to its premises (e.g. Some bananas are fruits. All fruits are pink.)
CONDITIONAL SYLLOGISMS
Another type of syllogism is called “conditional syllogism.” Just like the categorical syllogisms, they also have two premises and a conclusion. The difference is that the first premise has the form “If … then”. Syllogisms like this one are common in everyday life. Consider the following example from the story about Knut:
Premise 1: If it is raining, Knut’s wife needs an umbrella. Premise 2: It is raining.
Conclusion: Therefore, Knut’s wife needs an umbrella.
Conditional syllogisms are typically given in the abstract form: “If p then q”, where “p” is called the antecedent and “q” the consequent.
FORMS OF CONDITIONAL SYLLOGISMS
There are four major forms of conditional syllogisms: modus ponens, modus tollens, denying the antecedent, and affirming the consequent. These are illustrated in the table below (Figure 2) by means of the conditional syllogism above (i.e. If it is raining, Knut’s wife needs an umbrella). The table indicates the premises, the resulting conclusions and whether the form is valid. The bottom row displays the how frequently people correctly identify the validity of the syllogisms.
Figure 2. Different kinds of conditional syllogisms
Modus Ponens | Modus Tollens | Denying the Antecedent | Affirming the Consequent | |
Description | The antecedent of the first premise is affirmed in the second premise. | The consequent of the first premise is negated in the second premise. | The antecedent of the first premise is negated in the second premise. | The antecedent of the first premise is affirmed in the second premise. |
Formal |
If P then Q. P Therefore Q. |
If P then Q. Not-Q Therefore Not-P. |
If P then Q. Not-P Therefore Not-Q. |
If P then Q. Q Therefore P. |
Example |
If it is raining, Knut’s wife needs an umbrella. It is raining. Therefore Knut’s wife needs an umbrella. |
If it is raining, Knut’s wife needs an umbrella. Knut’s wife does not need an umbrella. Therefore it is not raining. |
If it is raining, Knut’s wife needs an umbrella. It is not raining. Therefore Knut’s wife does not need an umbrella. |
If it is raining, Knut’s wife needs an umbrella. Knut’s wife needs an umbrella. Therefore it is raining. |
Validity |
VALID |
VALID |
INVALID |
INVALID |
Correct Judgements |
97% correctly identify as valid. |
60% correctly identify as valid. |
40% correctly identify as invalid. |
40% correctly identify as invalid. |
As we can see, the validity of the syllogisms with valid conclusions is easier to judge correctly than the validity of the syllogisms with invalid conclusions. The conclusion in the instance of the modus ponens is apparently valid. In the example it is very clear that Knut’s wife needs an umbrella if it is raining.
The validity of the modus tollens is more difficult to recognize. Referring to the example, if Knut’s wife does not need an umbrella, it can’t be raining. The first premise says that if it is raining, she needs an umbrella. So, the reason for Knut’s wife not needing an umbrella is that it is not raining. Consequently, the conclusion is valid.
The validity of the remaining two kinds of conditional syllogisms is judged correctly by only 40% of people. If the method of denying the antecedent is applied, the second premise says that it is not raining. But from this fact it does not follow logically that Knut’s wife does not need an umbrella— she could need an umbrella for another reason, such as to shield from the sun.
Affirming the consequent in the case of the given example means that the second premise says that Knut’s wife needs an umbrella, but again the reason for this can be circumstances apart from rain. So, it does not logically follow that it is raining. Therefore, the conclusion of this syllogism is invalid.
The four kinds of syllogisms have shown that it is not always easy to make correct judgments concerning the validity of the conclusions. The following passages will deal with other errors people make during the process of conditional reasoning.
THE WASON SELECTION TASK
The Wason Selection Task is a famous experiment which shows that people make more reasoning errors in abstract situations than when the situation is taken from real life (Wason, 1966).
Figure 3.
In the abstract version of the Wason Selection Task, four cards are shown to the participants with a letter on one side and a number on the other (Figure 3). The task is to indicate the minimum number of cards that have to be turned over to test whether the following rule is observed: “If there is a vowel on one side then there is an even number on the other side.” 53% of participants selected the ‘E’ card which is correct, because turning this card over is necessary to test the truth of the rule. However another card still needs to be turned over. 64% indicated that the ‘4’ card has to be turned over which is not right. Only 4% of participants answered correctly that the ‘7’ card needs to be turned over in addition to the ‘E’. The correctness of turning over these two cards becomes more obvious if the same task is stated in terms of real-world items instead of vowels and numbers. One of the experiments for determining this was the beer/drinking-age problem used by Richard Griggs and James Cox (1982). This experiment is identical to the
Wason Selection Task except that instead of numbers and letters on the cards, everyday terms (beer, soda and ages) were used (Figure 4). Griggs and Cox gave the following rule to participants: “If a person is drinking beer then he or she must be older than 21.” In this case 73% of participants answered correctly, that the cards with “beer” and “14 years” have to be turned over to test whether the rule is kept.
Figure 4.
Why is the performance better in the case of real–world items?
There are two different approaches to explain why participants’ performance is significantly better in the case of the beer/drinking-age problem than in the abstract version of the Wason Selection Task: the permission schemas approach and the evolutionary approach.
The rule, “if a person is 21 years old or older then they are allowed to drink alcohol,” is well- known as an experience from everyday life. Based on a lifetime of learning rules in which one must satisfy some criteria for permission to perform a specific act, we have a permission schema already stored in long-term memory to think about such situations. Participants can apply this previously-learned permission schema to the Wason Selection Task for real–world items to improve participants’ performance. On the contrary such a permission schema from everyday life does not exist for the abstract version of the Wason Selection Task.
The evolutionary approach concerns the human ability of cheater detection . This approach states that an important aspect of human behavior across our evolutionary history is the ability for people to cooperate in a way that is mutually beneficial. As long as a person who receives a benefit also pays the relevant cost, everything works well in a social exchange. If someone cheats, however, and receives a benefit from others without paying the cost, problems arise. It is assumed that the ability to detect cheaters became a part of the human cognitive makeup during evolution. This cognitive ability improves the performance in the beer/drinking-age version of the Wason Selection Task as it allows people to detect a cheating person who does not behave according to the rule. Cheater-detection does not work in the case of the abstract version of the Wason Selection Task as vowels and numbers cannot behave in any way, much less cheat, and so the cheater detection mechanism is not activated.
INDUCTIVE REASONINGSo far we have discussed deductive reasoning, which is reaching conclusions based on logical rules applied to a set of premises. However, many problems cannot be represented in a way that would make it possible to use these rules to come to a conclusion. Inductive reasoning is the process of making observations and applying those observations via generalization to a different problem. Therefore one infers from a special case to the general principle, which is just the opposite of the procedure of deductive reasoning. A good example of inductive reasoning is the following:
Premise: All crows Knut and his wife have ever seen are black.
Conclusion: Therefore, they reason that all crows on earth are black.
In this example it is obvious that Knut and his wife infer from the simple observation about the crows they have seen to the general principle about all crows. Considering the figure above, this means that they infer from the subset (yellow circle) to the whole (blue circle). As with this example, it is typical in inductive reasoning that the premises are believed to support the conclusion, but do not ensure the conclusion.
Figure 6.
FO R M S O F I N D U C T I V E R E A S O N I N G
The two different forms of inductive reasoning are “strong” and “weak” induction. The former indicates that the truth of the conclusion is very likely if the assumed premises are true. An example for this form of reasoning is the one given in the previous section. In this case it is obvious that the premise (“All crows Knut and his wife have ever seen are black”) gives good evidence for the conclusion (“All crows on earth are black”) to be true.
Nevertheless it is still possible, although very unlikely, that not all crows are black.
On the contrary, conclusions reached by “weak induction” are supported by the premises in a relatively weak manner. In this approach, the truth of the premises makes the truth of the conclusion possible, but not likely. An example for this kind of reasoning is the following:
Premise: Knut always listens to music with his iPhone.
Conclusion: Therefore, he reasons that all music is only heard with iPhones.
In this instance the conclusion is obviously false. The information the premise contains is not very representative and although it is true, it does not give decisive evidence for the truth of the conclusion.
To sum it up, strong inductive reasoning yields conclusions which are very probable whereas the conclusions reached through weak inductive reasoning are unlikely to be true.
RELIABILITY OF CONCLUSIONS
If the strength of the conclusion of an inductive argument has to be determined, three factors concerning the premises play a decisive role. The of Knut and his wife’s observations about crows (see previous sections) displays these factors:
When Knut and his wife observe, in addition to the black crows in Germany, the crows in Spain, the number of observations they make concerning the crows increases. Furthermore, the representativeness of these observations is supported if Knut and his wife observe the crows at different times and in different places and see that they are black every time.
The quality of the evidence for all crows being black increases if Knut and his wife add scientific measurements that support the conclusion. For example, they could find out that the crows’ genes determine that the only color they can be is black.
Conclusions reached through a process of inductive reasoning are never definitely true, as no one has seen all crows on earth. It is possible, although very unlikely, that there is a green or brown exemplar. The three above factors contribute to the strength of an inductive argument. The stronger these factors are, the more reliable the conclusions reached through induction.
PROCESSES AND CONSTRAINTS
In a process of inductive reasoning, people often make use of certain heuristics. These heuristics often help people make adequate conclusions, but sometimes may cause errors. In the following sections, two of these heuristics (availability heuristic and representativeness heuristic) are explained. Subsequently, the confirmation bias is introduced, which can influence people to use their own opinions in reasoning without realizing it.
THE AVAILABILIT Y HEURISTIC
Things that are more easily remembered are judged to be more prevalent. An example of this is an experiment done by Lichtenstein et al. (1978).
Figure 5. Feminist bank tellers
People who are bank tellers:
People who are feminists and bank tellers:
Participants were asked to choose from a list which causes of death occur most often. Because of the availability heuristic people, judged more “spectacular” causes like homicide or tornadoes to cause more deaths than others, like asthma. The reason for this is that, for example, films and news in television are very often about spectacular and interesting causes of death. This is why this information is more readily available to the subjects in the experiment.
Another effect of the availability heuristic is called illusory correlations. People tend to judge according to stereotypes. It seems to them that there are correlations between certain events which in reality do not exist— this is what is known as “prejudice.” Usually a correlation seems to exist between negative features and a certain class of people. If, for example, one’s neighbor is jobless and very lazy one tends to correlate these two attributes and create the prejudice that all jobless people are lazy. This illusory correlation occurs because one takes into account information which is available and judges this to be prevalent in many cases.
THE REPRESENTATIVENESS HEURISTIC
If people have to judge the probability of an event, they try to find a comparable event and assume that the two events have a similar probability. Amos Tversky and Daniel Kahneman (1974) presented the following task to their participants in an experiment: “We randomly chose a man from the population of the U.S., Robert, who wears glasses, speaks quietly and reads a lot. Is it more likely that he is a librarian or a farmer?” More of the participants answered that Robert is a librarian, which is an effect of the representativeness heuristic.
Participants compared the description of Robert with the typical depiction of a librarian, and found that the description was more like a librarian than a farmer. So, the event of a typical librarian is more comparable with Robert than the event of a typical farmer. Of course this effect may lead to errors, as Robert is randomly chosen from the population and as it is perfectly possible that he is a farmer even though he speaks quietly and wears glasses.
The representativeness heuristic also leads to errors in reasoning in cases where the conjunction rule is violated. This rule states that the conjunction of two events is never more likely to be the case than the single events alone. An example for this is the case of the feminist bank teller (Tversky & Kahneman, 1983). If we are introduced to a woman who is very interested in women’s rights and has participated in many political activities in college, and we are to decide whether it is more likely that she is a bank teller or a feminist bank teller, we are drawn to conclude the latter as the facts we have learned about her resemble the event of a feminist bank teller more than the event of only being a bank teller.
Figure 6. Deductive and inductive reasoning
However, it is in fact more likely that somebody is just a bank teller than it is that someone is a feminist in addition to being a bank teller. This effect is illustrated in Figure 5, where the green square, which stands for just being a bank teller, is much larger and thus more probable than the smaller orange square, which displays the conjunction of bank tellers and feminists, which is a subset of bank tellers.
CONFIRMATION BIAS
People tend to use what they believe to be true or good to when using evidence to make inferences. If, for example, someone believes that they have bad luck on Friday the thirteenth, they will especially look for every negative event on this particular date but will be inattentive to negative events on other days. This behavior strengthens the belief that there exists a relationship between Friday the thirteenth and having bad luck. This example shows that all information is not equally taken into account to come to a conclusion, but rather on seeks out information which supports one’s own belief. This effect leads to errors as people tend to reason in a subjective manner, if personal interests and beliefs are involved.
All the mentioned factors influence the subjective probability of an event so that it differs from the actual probability (probability heuristic). Of course all of these factors do not always appear alone, but they influence one another and can occur in combination during the process of reasoning.
INDUCTION VS DEDUCTION
The table below (Figure 7) summarizes the most prevalent properties and differences between deductive and inductive reasoning which are important to keep in mind.
Figure 7. Induction vs. deduction
Deductive Reasoning | Inductive Reasoning | |
Premises | Stated as facts or general principles (“It is warm in the Summer in Spain.”) | Based on observations of specific cases (“All crows Knut and his wife have seen are black.”) |
Conclusion |
Conclusion is more special than the information the premises provide. It is reached directly by applying logical rules to the premises. |
Conclusion is more general than the information the premises provide. It is reached by generalizing the premises’ information. |
Validity |
If the premises are true, the conclusion must be true. |
If the premises are true, the conclusion is probably true. |
Usage |
More difficult to use (mainly in logical problems). One needs facts which are definitely true. |
Used often in everyday life (fast and easy). Evidence is used instead of proved facts. |
DECISION MAKINGThe psychological process of decision making is critical in everyday daily life. Imagine Knut deciding between packing more blue or more green shirts for his vacation (which would only have minor consequences) but also about applying a specific job or having children with his wife (which would have important consequences in his future life).
There are three different ways to analyze decision making. The normative approach assumes a rational decision-maker with well-defined preferences. The descriptive approach is based on empirical observations and on experimental studies of choice behavior. The prescriptive approach develops methods to improve decision making.
MISLEADING EFFECTS
People may struggle to make the “right” decision because of different misleading effects, which mainly arise because of the constraints of inductive reasoning. In general this means that our model of a situation or problem might not be ideal to solve it in an optimal way.
One misleading effect is the so-called focusing illusion. By considering only the most obvious aspects of a situation to make a decision, people often neglect more important aspects. For example, in considering job offers in different locations, a person may pay too much attention to salient aspects of a location such as weather, and relatively less to highly important aspects such as circumstances at work.
The way a problem is framed can evoke different decision strategies. If a problem is framed in terms of gains, people tend to use a risk-aversion strategy, while framing a problem in terms of losses leads to a risk-taking strategy. An example of the same problem and predictably different choices is the following experiment: A group of people is asked to imagine themselves $300 richer than they are. They are then confronted with the choice of a sure gain of $100 or an equal chance to gain $200 or nothing. Most people avoid the risk and take the sure gain, which means they take the risk-aversion strategy. Alternatively, if people are asked to assume themselves to be $500 richer than in reality, given the options of a sure loss of $100 or an equal chance to lose $200 or nothing, the majority opts for the risk of losing $200, which represents a risk-taking strategy. This phenomenon is known as a framing effect and can also be illustrated by figure 8 above, which shows a concave function for gains and a convex one for losses. (Foundations of Cognitive Psychology, Levitin, D. J., 2002)
Figure 8. Relation between (monetary) gains/losses and their subjective value according to Prospect Theory
Social Cognition and Decision-Making
Imagine you are walking toward your classroom and you see your teacher and a fellow student you know to be disruptive in class whispering together in the hallway. As you approach, both of them quit talking, nod to you, and then resume their urgent whispers after you pass by. What would you make of this scene? What story might you tell yourself to help explain this interesting and unusual behavior?
People know intuitively that we can better understand others’ behavior if we know the thoughts contributing to the behavior. In this example, you might guess that your teacher harbors several concerns about the disruptive student, and therefore you believe their whispering is related to this. The area of psychology that focuses on how people think about others and about the social world is called social cognition.
Researchers of social cognition study how people make sense of themselves and others to make judgments, form attitudes, and make predictions about the future. Much of the research in social cognition has demonstrated that humans are adept at distilling large amounts of information into smaller, more usable chunks, and that we possess many cognitive tools that allow us to efficiently navigate our environments. This research has also illuminated many social factors that can influence these judgments and predictions. Not only can our past experiences, expectations, motivations, and moods impact our reasoning, but many of our decisions and behaviors are driven by unconscious processes and implicit attitudes we are unaware of having. The goal of this module is to highlight the mental tools we use to navigate and make sense of our complex social world, and describe some of the emotional, motivational, and cognitive factors that affect our reasoning.
Simplifying Our Social World
Consider how much information you come across on any given day; just looking around your bedroom, there are hundreds of objects, smells, and sounds. How do we simplify all this information to attend to what is important and make decisions quickly and efficiently? In part, we do it by forming schemas of the various people, objects, situations, and events we encounter. A schema is a mental model, or representation, of any of the various things we come across in our daily lives. A schema (related to the word schematic) is kind of like a mental blueprint for how we expect something to be or behave. It is an organized body of general information or beliefs we develop from direct encounters, as well as from secondhand sources. Rather than spending copious amounts of time learning about each new individual object (e.g., each new dog we see), we rely on our schemas to tell us that a newly encountered dog probably barks, likes to fetch, and enjoys treats. In this way, our schemas greatly reduce the amount of cognitive work we need to do and allow us to “go beyond the information given” (Bruner, 1957).
We can hold schemas about almost anything—individual people (person schemas), ourselves (self-schemas), and recurring events (event schemas, or scripts). Each of these types of schemas is useful in its own way. For example, event schemas allow us to navigate new situations efficiently and seamlessly. A script for dining at a restaurant would indicate that one should wait to be seated by the host or hostess, that food should be ordered from a menu, and that one is expected to pay the check at the end of the meal. Because the majority of dining situations conform to this general format, most diners just need to follow their mental scripts to know what to expect and how they should behave, greatly reducing their cognitive workload.
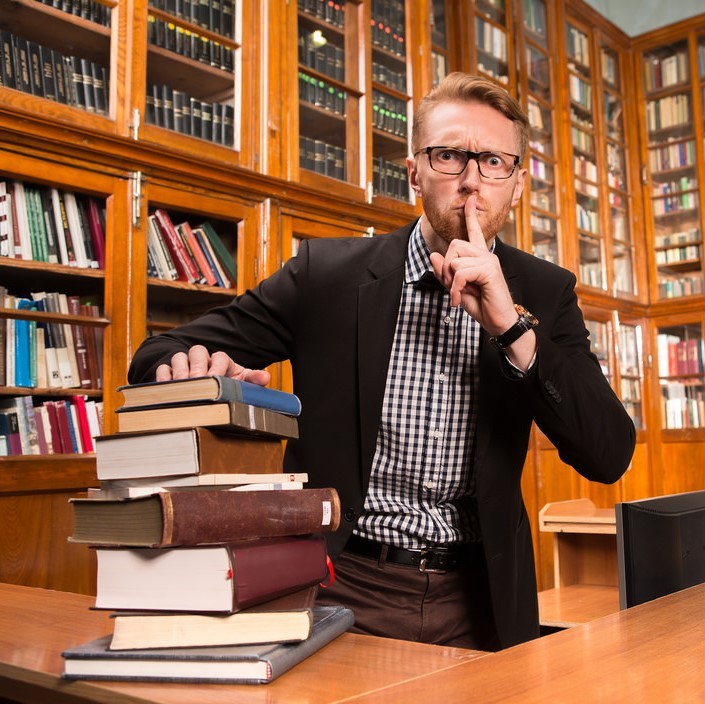
Another important way we simplify our social world is by employing heuristics, which are mental shortcuts that reduce complex problem-solving to more simple, rule-based decisions. For example, have you ever had a hard time trying to decide on a book to buy, then you see one ranked highly on a book review website? Although selecting a book to purchase can be a complicated decision, you might rely on the “rule of thumb” that a recommendation from a credible source is likely a safe bet—so you buy it. A common instance of using heuristics is when people are faced with judging whether an object belongs to a particular category. For example, you would easily classify a pit bull into the category of “dog.” But what about a coyote? Or a fox? A plastic toy dog? In order to make this classification (and many others), people may rely on the representativeness heuristic to arrive at a quick decision (Kahneman & Tversky, 1972, 1973). Rather than engaging in an in-depth consideration of the object’s attributes, one can simply judge the likelihood of the object belonging to a category, based on how similar it is to one’s mental representation of that category. For example, a perceiver may quickly judge a female to be an athlete based on the fact that the female is tall, muscular, and wearing sports apparel—which fits the perceiver’s representation of an athlete’s characteristics.
In many situations, an object’s similarity to a category is a good indicator of its membership in that category, and an individual using the representativeness heuristic will arrive at a correct judgment. However, when base-rate information (e.g., the actual percentage of athletes in the area and therefore the probability that this person actually is an athlete) conflicts with representativeness information, use of this heuristic is less appropriate. For example, if asked to judge whether a quiet, thin man who likes to read poetry is a classics professor at a prestigious university or a truck driver, the representativeness heuristic might lead one to guess he’s a professor. However, considering the base-rates, we know there are far fewer university classics professors than truck drivers. Therefore, although the man fits the mental image of a professor, the actual probability of him being one (considering the number of professors out there) is lower than that of being a truck driver.
In addition to judging whether things belong to particular categories, we also attempt to judge the likelihood that things will happen. A commonly employed heuristic for making this type of judgment is called the availability heuristic. People use the availability heuristic to evaluate the frequency or likelihood of an event based on how easily instances of it come to mind (Tversky & Kahneman, 1973). Because more commonly occurring events are more likely to be cognitively accessible (or, they come to mind more easily), use of the availability heuristic can lead to relatively good approximations of frequency. However, the heuristic can be less reliable when judging the frequency of relatively infrequent but highly accessible events. For example, do you think there are more words that begin with “k,” or more that have “k” as the third letter? To figure this out, you would probably make a list of words that start with “k” and compare it to a list of words with “k” as the third letter. Though such a quick test may lead you to believe there are more words that begin with “k,” the truth is that there are 3 times as many words that have “k” as the third letter (Schwarz et al., 1991). In this case, words beginning with “k” are more readily available to memory (i.e., more accessible), so they seem to be more numerous. Another example is the very common fear of flying: dying in a plane crash is extremely rare, but people often overestimate the probability of it occurring because plane crashes tend to be highly memorable and publicized.
In summary, despite the vast amount of information we are bombarded with on a daily basis, the mind has an entire kit of “tools” that allows us to navigate that information efficiently. In addition to category and frequency judgments, another common mental calculation we perform is predicting the future. We rely on our predictions about the future to guide our actions. When deciding what entrée to select for dinner, we may ask ourselves, “How happy will I be if I choose this over that?” The answer we arrive at is an example of a future prediction. In the next section, we examine individuals’ ability to accurately predict others’ behaviors, as well as their own future thoughts, feelings, and behaviors, and how these predictions can impact their decisions.
Making Predictions About the Social World
Whenever we face a decision, we predict our future behaviors or feelings in order to choose the best course of action. If you have a paper due in a week and have the option of going out to a party or working on the paper, the decision of what to do rests on a few things: the amount of time you predict you will need to write the paper, your prediction of how you will feel if you do poorly on the paper, and your prediction of how harshly the professor will grade it.
In general, we make predictions about others quickly, based on relatively little information. Research on “thin-slice judgments” has shown that perceivers are able to make surprisingly accurate inferences about another person’s emotional state, personality traits, and even sexual orientation based on just snippets of information—for example, a 10-second video clip (Ambady, Bernieri, & Richeson, 2000; Ambady, Hallahan, & Conner, 1999; Ambady & Rosenthal, 1993). Furthermore, these judgments are predictive of the target’s future behaviors. For example, one study found that students’ ratings of a teacher’s warmth, enthusiasm, and attentiveness from a 30-second video clip strongly predicted that teacher’s final student evaluations after an entire semester (Ambady & Rosenthal, 1993). As might be expected, the more information there is available, the more accurate many of these judgments become (Carney, Colvin, & Hall, 2007).
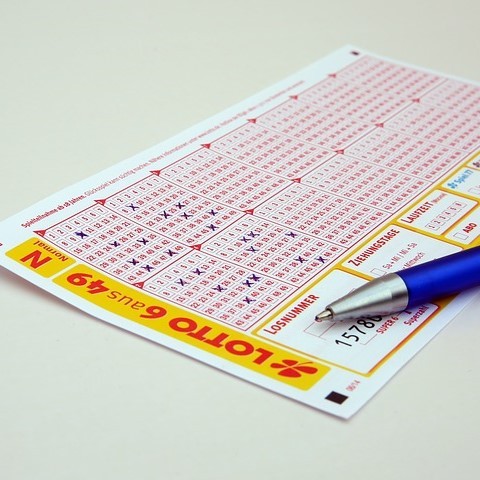
Because we seem to be fairly adept at making predictions about others, one might expect predictions about the self to be foolproof, given the considerable amount of information one has about the self compared to others. To an extent, research has supported this conclusion. For example, our own predictions of our future academic performance are more accurate than peers’ predictions of our performance, and self-expressed interests better predict occupational choice than career inventories (Shrauger & Osberg, 1981). Yet, it is not always the case that we hold greater insight into ourselves. While our own assessment of our personality traits does predict certain behavioral tendencies better than peer assessment of our personality, for certain behaviors, peer reports are more accurate than self-reports (Kolar, Funder, & Colvin, 1996; Vazire, 2010). Similarly, although we are generally aware of our knowledge, abilities, and future prospects, our perceptions are often overly positive, and we display overconfidence in their accuracy and potential (Metcalfe, 1998). For example, we tend to underestimate how much time it will take us to complete a task, whether it is writing a paper, finishing a project at work, or building a bridge—a phenomenon known as the planning fallacy (Buehler, Griffin, & Ross, 1994). The planning fallacy helps explain why so many college students end up pulling all-nighters to finish writing assignments or study for exams. The tasks simply end up taking longer than expected. On the positive side, the planning fallacy can also lead individuals to pursue ambitious projects that may turn out to be worthwhile. That is, if they had accurately predicted how much time and work it would have taken them, they may have never started it in the first place.
The other important factor that affects decision-making is our ability to predict how we will feel about certain outcomes. Not only do we predict whether we will feel positively or negatively, we also make predictions about how strongly and for how long we will feel that way. Research demonstrates that these predictions of one’s future feelings—known as affective forecasting—are accurate in some ways but limited in others (Gilbert & Wilson, 2007). We are adept at predicting whether a future event or situation will make us feel positively or negatively (Wilson & Gilbert, 2003), but we often incorrectly predict the strength or duration of those emotions. For example, you may predict that if your favorite sports team loses an important match, you will be devastated. Although you’re probably right that you will feel negative (and not positive) emotions, will you be able to accurately estimate how negative you’ll feel? What about how long those negative feelings will last?
Predictions about future feelings are influenced by the impact bias : the tendency for a person to overestimate the intensity of their future feelings. For example, by comparing people’s estimates of how they expected to feel after a specific event to their actual feelings after the event, research has shown that people generally overestimate how badly they will feel after a negative event—such as losing a job—and they also overestimate how happy they will feel after a positive event—such as winning the lottery (Brickman, Coates, & Janoff-Bullman, 1978). Another factor in these estimations is the durability bias. The durability bias refers to the tendency for people to overestimate how long (or, the duration) positive and negative events will affect them. This bias is much greater for predictions regarding negative events than positive events, and occurs because people are generally unaware of the many psychological mechanisms that help us adapt to and cope with negative events (Gilbert, Pinel, Wilson, Blumberg, & Wheatley, 1998;Wilson, Wheatley, Meyers, Gilbert, & Axsom, 2000).
In summary, individuals form impressions of themselves and others, make predictions about the future, and use these judgments to inform their decisions. However, these judgments are shaped by our tendency to view ourselves in an overly positive light and our inability to appreciate our habituation to both positive and negative events. In the next section, we will discuss how motivations, moods, and desires also shape social judgment.
Hot Cognition: The Influence of Motivations, Mood, and Desires on Social Judgment
Although we may believe we are always capable of rational and objective thinking (for example, when we methodically weigh the pros and cons of two laundry detergents in an unemotional—i.e., “cold”—manner), our reasoning is often influenced by our motivations and mood. Hot cognition refers to the mental processes that are influenced by desires and feelings. For example, imagine you receive a poor grade on a class assignment. In this situation, your ability to reason objectively about the quality of your assignment may be limited by your anger toward the teacher, upset feelings over the bad grade, and your motivation to maintain your belief that you are a good student. In this sort of scenario, we may want the situation to turn out a particular way or our belief to be the truth. When we have these directional goals, we are motivated to reach a particular outcome or judgment and do not process information in a cold, objective manner.
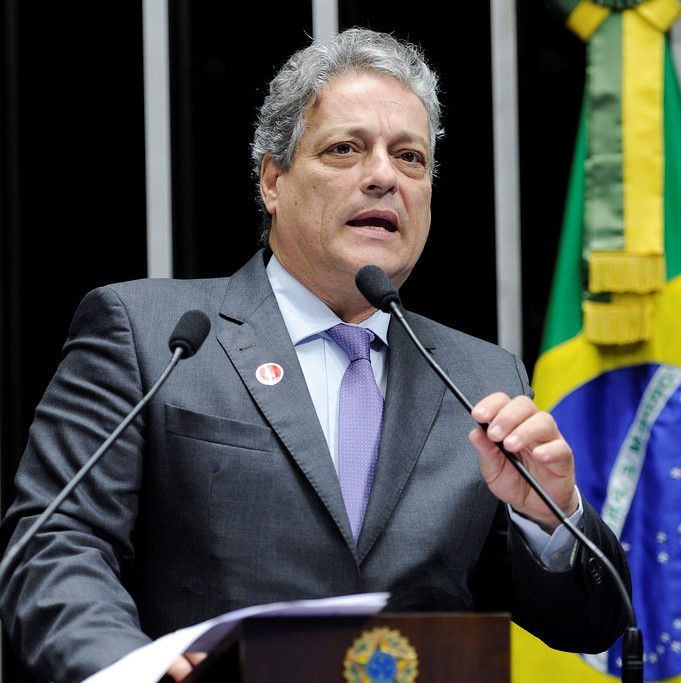
Directional goals can bias our thinking in many ways, such as leading to motivated skepticism, whereby we are skeptical of evidence that goes against what we want to believe despite the strength of the evidence (Ditto & Lopez, 1992). For example, individuals trust medical tests less if the results suggest they have a deficiency compared to when the results suggest they are healthy. Through this motivated skepticism, people often continue to believe what they want to believe, even in the face of nearly incontrovertible evidence to the contrary.
There are also situations in which we do not have wishes for a particular outcome but our goals bias our reasoning, anyway. For example, being motivated to reach an accurate conclusion can influence our reasoning processes by making us more cautious—leading to indecision. In contrast, sometimes individuals are motivated to make a quick decision, without being particularly concerned about the quality of it. Imagine trying to choose a restaurant with a group of friends when you’re really hungry. You may choose whatever’s nearby without caring if the restaurant is the best or not. This need for closure (the desire to come to a firm conclusion) is often induced by time constraints (when a decision needs to be made quickly) as well as by individual differences in the need for closure (Webster & Kruglanski, 1997). Some individuals are simply more uncomfortable with ambiguity than others, and are thus more motivated to reach clear, decisive conclusions.
Just as our goals and motivations influence our reasoning, our moods and feelings also shape our thinking process and ultimate decisions. Many of our decisions are based in part on our memories of past events, and our retrieval of memories is affected by our current mood. For example, when you are sad, it is easier to recall the sad memory of your dog’s death than the happy moment you received the dog. This tendency to recall memories similar in valence to our current mood is known as mood-congruent memory (Blaney, 1986; Bower 1981, 1991; DeSteno, Petty, Wegener, & Rucker, 2000; Forgas, Bower, & Krantz, 1984; Schwarz, Strack, Kommer, & Wagner, 1987). The mood we were in when the memory was recorded becomes a retrieval cue; our present mood primes these congruent memories, making them come to mind more easily (Fiedler, 2001). Furthermore, because the availability of events in our memory can affect their perceived frequency (the availability heuristic), the biased retrieval of congruent memories can then impact the subsequent judgments we make (Tversky & Kahneman, 1973). For example, if you are retrieving many sad memories, you might conclude that you have had a tough, depressing life.
In addition to our moods influencing the specific memories we retrieve, our moods can also influence the broader judgments we make. This sometimes leads to inaccuracies when our current mood is irrelevant to the judgment at hand. In a classic study demonstrating this effect, researchers found that study participants rated themselves as less-satisfied with their lives in general if they were asked on a day when it happened to be raining vs. sunny (Schwarz & Clore, 1983). However, this occurred only if the participants were not aware that the weather might be influencing their mood. In essence, participants were in worse moods on rainy days than sunny days, and, if unaware of the weather’s effect on their mood, they incorrectly used their mood as evidence of their overall life satisfaction.
In summary, our mood and motivations can influence both the way we think and the decisions we ultimately make. Mood can shape our thinking even when the mood is irrelevant to the judgment, and our motivations can influence our thinking even if we have no particular preference about the outcome. Just as we might be unaware of how our reasoning is influenced by our motives and moods, research has found that our behaviors can be determined by unconscious processes rather than intentional decisions, an idea we will explore in the next section.
Automaticity
Do we actively choose and control all our behaviors or do some of these behaviors occur automatically? A large body of evidence now suggests that many of our behaviors are, in fact, automatic. A behavior or process is considered automatic if it is unintentional, uncontrollable, occurs outside of conscious awareness, or is cognitively efficient (Bargh & Chartrand, 1999). A process may be considered automatic even if it does not have all these features; for example, driving is a fairly automatic process, but is clearly intentional. Processes can become automatic through repetition, practice, or repeated associations. Staying with the driving example: although it can be very difficult and cognitively effortful at the start, over time it becomes a relatively automatic process, and aspects of it can occur outside conscious awareness.
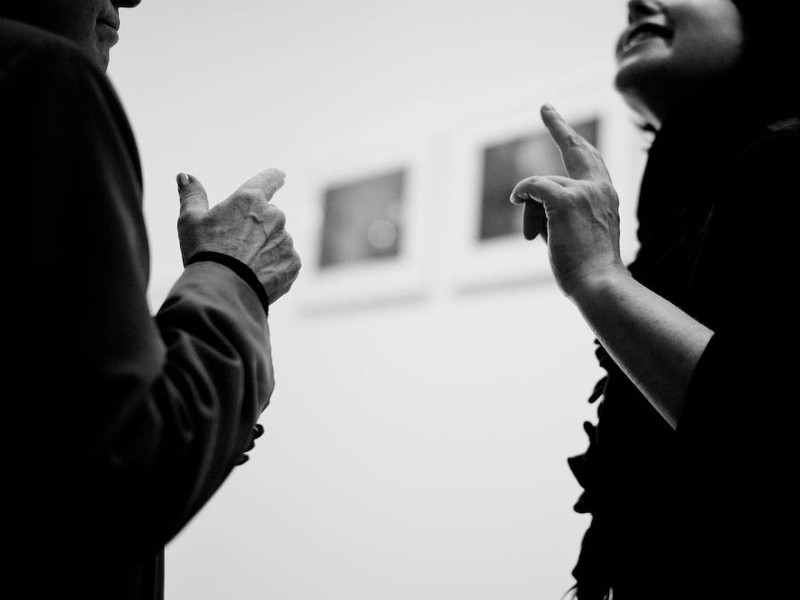
In addition to practice leading to the learning of automatic behaviors, some automatic processes, such as fear responses, appear to be innate. For example, people quickly detect negative stimuli, such as negative words, even when those stimuli are presented subliminally (Dijksterhuis & Aarts, 2003; Pratto & John, 1991). This may represent an evolutionarily adaptive response that makes individuals more likely to detect danger in their environment. Other innate automatic processes may have evolved due to their pro-social outcomes. The chameleon effect—where individuals nonconsciously mimic the postures, mannerisms, facial expressions, and other behaviors of their interaction partners—is an example of how people may engage in certain behaviors without conscious intention or awareness (Chartrand & Bargh, 1999). For example, have you ever noticed that you’ve picked up some of the habits of your friends? Over time, but also in brief encounters, we will nonconsciously mimic those around us because of the positive social effects of doing so. That is, automatic mimicry has been shown to lead to more positive social interactions and to increase liking between the mimicked person and the mimicking person.
When concepts and behaviors have been repeatedly associated with each other, one of them can be primed—i.e., made more cognitively accessible—by exposing participants to the (strongly associated) other one. For example, by presenting participants with the concept of a doctor, associated concepts such as “nurse” or “stethoscope” are primed. As a result, participants recognize a word like “nurse” more quickly (Meyer, & Schvaneveldt, 1971). Similarly, stereotypes can automatically prime associated judgments and behaviors. Stereotypes are our general beliefs about a group of people and, once activated, they may guide our judgments outside of conscious awareness. Similar to schemas, stereotypes involve a mental representation of how we expect a person will think and behave. For example, someone’s mental schema for women may be that they’re caring, compassionate, and maternal; however, a stereotype would be that all women are examples of this schema. As you know, assuming all people are a certain way is not only wrong but insulting, especially if negative traits are incorporated into a schema and subsequent stereotype.
In a now classic study, Patricia Devine (1989) primed study participants with words typically associated with Black people (e.g., “blues,” “basketball”) in order to activate the stereotype of Black people. Devine found that study participants who were primed with the Black stereotype judged a target’s ambiguous behaviors as being more hostile (a trait stereotypically associated with Black people) than nonprimed participants. Research in this area suggests that our social context—which constantly bombards us with concepts—may prime us to form particular judgments and influence our thoughts and behaviors.
In summary, there are many cognitive processes and behaviors that occur outside of our awareness and despite our intentions. Because automatic thoughts and behaviors do not require the same level of cognitive processing as conscious, deliberate thinking and acting, automaticity provides an efficient way for individuals to process and respond to the social world. However, this efficiency comes at a cost, as unconsciously held stereotypes and attitudes can sometimes influence us to behave in unintended ways. We will discuss the consequences of both consciously and unconsciously held attitudes in the next section.
Conclusion
Decades of research on social cognition have revealed many of the “tricks” and “tools” we use to efficiently process the limitless amounts of social information we encounter. These tools are quite useful for organizing that information to arrive at quick decisions. When you see an individual engage in a behavior, such as seeing a man push an elderly woman to the ground, you form judgments about his personality, predictions about the likelihood of him engaging in similar behaviors in the future, as well as predictions about the elderly woman’s feelings and how you would feel if you were in her position. As the research presented in this chapter demonstrates, we are adept and efficient at making these judgments and predictions, but they are not made in a vacuum. Ultimately, our perception of the social world is a subjective experience, and, consequently, our decisions are influenced by our experiences, expectations, emotions, motivations, and current contexts. Being aware of when our judgments are most accurate, and how our judgments are shaped by social influences, prepares us to be in a much better position to appreciate, and potentially counter, their effects.
Vocabulary
- Affective forecasting
- Predicting how one will feel in the future after some event or decision.
- Attitude
- A psychological tendency that is expressed by evaluating a particular entity with some degree of favor or disfavor.
- Automatic
- A behavior or process has one or more of the following features: unintentional, uncontrollable, occurring outside of conscious awareness, and cognitively efficient.
- Availability heuristic
- A heuristic in which the frequency or likelihood of an event is evaluated based on how easily instances of it come to mind.
- Chameleon effect
- The tendency for individuals to nonconsciously mimic the postures, mannerisms, facial expressions, and other behaviors of one’s interaction partners.
- Directional goals
- The motivation to reach a particular outcome or judgment.
- Durability bias
- A bias in affective forecasting in which one overestimates for how long one will feel an emotion (positive or negative) after some event.
- Evaluative priming task
- An implicit attitude task that assesses the extent to which an attitude object is associated with a positive or negative valence by measuring the time it takes a person to label an adjective as good or bad after being presented with an attitude object.
- Explicit attitude
- An attitude that is consciously held and can be reported on by the person holding the attitude.
- Heuristics
- A mental shortcut or rule of thumb that reduces complex mental problems to more simple rule-based decisions.
- Hot cognition
- The mental processes that are influenced by desires and feelings.
- Impact bias
- A bias in affective forecasting in which one overestimates the strength or intensity of emotion one will experience after some event.
- Implicit Association Test
- An implicit attitude task that assesses a person’s automatic associations between concepts by measuring the response times in pairing the concepts.
- Implicit attitude
- An attitude that a person cannot verbally or overtly state.
- Implicit measures of attitudes
- Measures of attitudes in which researchers infer the participant’s attitude rather than having the participant explicitly report it.
- Mood-congruent memory
- The tendency to be better able to recall memories that have a mood similar to our current mood.
- Motivated skepticism
- A form of bias that can result from having a directional goal in which one is skeptical of evidence despite its strength because it goes against what one wants to believe.
- Need for closure
- The desire to come to a decision that will resolve ambiguity and conclude an issue.
- Planning fallacy
- A cognitive bias in which one underestimates how long it will take to complete a task.
- Primed
- A process by which a concept or behavior is made more cognitively accessible or likely to occur through the presentation of an associated concept.
- Representativeness heuristic
- A heuristic in which the likelihood of an object belonging to a category is evaluated based on the extent to which the object appears similar to one’s mental representation of the category.
- Schema
- A mental model or representation that organizes the important information about a thing, person, or event (also known as a script).
- The study of how people think about the social world.
- Stereotypes
- Our general beliefs about the traits or behaviors shared by group of people.
References
- Ambady, N., & Rosenthal, R. (1993). Half a minute: Predicting teacher evaluations from thin slices of nonverbal behavior and physical attractiveness. Journal of Personality and Social Psychology, 64, 431–441.
- Ambady, N., Bernieri, F. J., & Richeson, J. A. (2000). Toward a histology of social behavior: Judgmental accuracy from thin slices of the behavioral stream. Advances in Experimental Social Psychology, 32, 201–271. San Diego, CA: Academic Press.
- Ambady, N., Hallahan, M., & Conner, B. (1999). Accuracy of judgments of sexual orientation from thin slices of behavior. Journal of Personality and Social Psychology, 77, 538–547.
- Bargh, J. A., & Chartrand, T. L. (1999). The unbearable automaticity of being. American Psychologist, 54, 462–479.
- Blaney, P. H. (1986). Affect and memory: A review. Psychological Bulletin, 99, 229–246.
- Bower, G. H. (1991). Mood congruity of social judgments. In J. P. Forgas (Ed.), Emotion and social judgments (pp. 31–53). New York, NY: Pergamon.
- Bower, G. H. (1981). Mood and memory. American Psychologist, 36, 129–148.
- Brickman, P., Coates, D., & Janoff-Bullman, R. (1978). Lottery winners and accident victims: Is happiness relative? Journal of Personality and Social Psychology, 36, 917–927.
- Bruner, J. S. (1957). Going beyond the information given. In J. S. Bruner, E. Brunswik, L. Festinger, F. Heider, K. F. Muenzinger, C. E. Osgood, & D. Rapaport, (Eds.), Contemporary approaches to cognition (pp. 41–69). Cambridge, MA: Harvard University Press.
- Buehler, R., Griffin, D., & Ross, M. (1994). Exploring the “planning fallacy”: Why people underestimate their task completion times. Journal of Personality and Social Psychology, 67, 366–381.
- Carney, D. R., Colvin, C. R., & Hall, J. A. (2007). A thin slice perspective on the accuracy of first impressions. Journal of Research in Personality, 41, 1054–1072.
- Chartrand, T. L., & Bargh, J. A. (1999). The chameleon effect: The perception-behavior link and social interaction. Journal of Personality and Social Psychology, 76, 893–910.
- DeSteno, D., Petty, R., Wegener, D., & Rucker, D. (2000). Beyond valence in the perception of likelihood: The role of emotion specificity. Journal of Personality and Social Psychology, 78, 397–416.
- Devine, P. (1989). Stereotypes and prejudice: Their automatic and controlled components. Journal of Personality and Social Psychology, 5, 5–18.
- Dijksterhuis, A., & Aarts, H. (2003). On wildebeests and humans: The preferential detection of negative stimuli. Psychological Science, 14, 14–18.
- Ditto, P. H., & Lopez, D. F. (1992). Motivated skepticism: Use of differential decision criteria for preferred and nonpreferred conclusions. Journal of Personality and Social Psychology, 63, 568–584.
- Eagly, A. H., & Chaiken, S. (1993). The psychology of attitudes (p. 1). Fort Worth, TX: Harcourt Brace Jovanovich College Publishers.
- Fazio, R. H., & Olson, M. A. (2003). Implicit measures in social cognition research: Their meaning and use. Annual Review of Psychology, 54, 297–327.
- Fazio, R. H., Jackson, J. R., Dunton, B. C., & Williams, C. J. (1995). Variability in automatic activation as an unobtrusive measure of racial attitudes: A bona fide pipeline? Journal of Personality and Social Psychology, 69, 1013–1027.
- Fiedler, K. (2001). Affective influences on social information processing. In J. P. Forgas (Ed.), Handbook of affect and social cognition (pp. 163–185). Mahwah, NJ: Lawrence Erlbaum Associates.
- Forgas, J. P., Bower, G. H., & Krantz, S. (1984). The influence of mood on perceptions of social interactions. Journal of Experimental Social Psychology, 20, 497–513.
- Gilbert, D. T., & Wilson, T. D. (2007). Prospection: Experiencing the future. Science, 317, 1351–1354.
- Gilbert, D. T., Pinel, E. C., Wilson, T. D., Blumberg, S. J., & Wheatley, T. P. (1998). Immune neglect: A source of durability bias in affective forecasting. Journal of Personality and Social Psychology, 75, 617–638.
- Greenwald, A. G., & Banaji, M. R. (1995). Implicit social cognition: Attitudes, self-esteem, and stereotypes. Psychological Review, 102, 4–27.
- Greenwald, A. G., McGhee, D. E., & Schwartz, J. K. L. (1998). Measuring individual differences in implicit cognition: The implicit association test. Journal of Personality and Social Psychology, 74, 1464–1480.
- Kahneman, D., & Tversky, A. (1973). On the psychology of prediction. Psychological Review, 80, 237–251.
- Kahneman, D., & Tversky, A. (1972). Subjective probability: A judgment of representativeness. Cognitive Psychology, 3, 430–454.
- Kolar, D. W., Funder, D. C., & Colvin, C. R. (1996). Comparing the accuracy of personality judgments by the self and knowledgeable others. Journal of Personality, 64, 311–337.
- Likert, R. (1932). A technique for the measurement of attitudes. Archives of Psychology, 140, 1–55.
- McConnell, A. R., & Leibold, J. M. (2001). Relations among the implicit association test, discriminatory behavior, and explicit measures of racial attitudes. Journal of Experimental Social Psychology, 37, 435–442.
- Metcalfe, J. (1998). Cognitive optimism: Self-deception or memory-based processing heuristics? Personality and Social Psychology Review, 2, 100–110.
- Meyer, D. E., & Schvaneveldt, R. W. (1971). Facilitation in recognizing pairs of words: Evidence of a dependence between retrieval operations. Journal of Experimental Psychology, 90, 227–234.
- Nosek, B. A., Banaji, M., & Greenwald, A. G. (2002). Harvesting implicit group attitudes and beliefs from a demonstration website. Group Dynamics: Theory, Research, and Practice, 6, 101–115.
- Osgood, C. E., Suci, G., & Tannenbaum, P. (1957). The measurement of meaning. Urbana, IL: University of Illinois Press.
- Payne, B. K. (2001). Prejudice and perception: The role of automatic and controlled processes in misperceiving a weapon. Journal of Personality and Social Psychology, 81, 181–192.
- Pratto, F., & John, O. P. (1991). Automatic vigilance: The attention-grabbing power of negative social information. Journal of Personality and Social Psychology, 61, 380–391.
- Schwarz, N., & Clore, G. L. (1983). Mood, misattribution, and judgments of well-being: Informative and directive functions of affective states. Journal of Personality and Social Psychology, 45, 513–523.
- Schwarz, N., Bless, H., Strack, F., Klumpp, G., Rittenauer-Schatka, H., & Simons, A. (1991). Ease of retrieval as information: Another look at the availability heuristic. Journal of Personality and Social Psychology, 61(2), 195.
- Schwarz, N., Strack, F., Kommer, D., & Wagner, D. (1987). Soccer, rooms, and the quality of your life: Mood effects on judgments of satisfaction with life in general and with specific domains. Journal of Social Psychology, 17, 69–79.
- Shrauger, J. S., & Osberg, T. M. (1981). The relative accuracy of self-predictions and judgments by others in psychological assessment. Psychological Bulletin, 90, 322–351.
- Tversky, A., & Kahneman, D. (1973). Availability: A heuristic for judging frequency and probability. Cognitive Psychology, 5, 207–232.
- Vazire, S. (2010). Who knows what about a person? The self-other asymmetry (SOKA) model. Journal of Personality and Social Psychology, 98, 281–300.
- Webster, D. M., & Kruglanski, A. W. (1997). Cognitive and social consequences of the need for cognitive closure. European Review of Social Psychology, 18, 133–173.
- Wilson, T. D., & Gilbert, D. T. (2003). Affective forecasting. Advances in Experimental Social Psychology, 35, 345–411.
- Wilson, T. D., Wheatley, T. P., Meyers, J. M., Gilbert, D. T., & Axsom, D. (2000). Focalism: A source of durability bias in affective forecasting. Journal of Personality and Social Psychology, 78, 821–836.
REFERENCES
_________________________________________________________________________________________________________________________________________________________
Krawczyk,Daniel (2018). Reasoning: The Neuroscience of How We Think. Elsevier.
Goldstein, E. Bruce (2005). Cognitive Psychology – Connecting, Mind Research, and Everyday Experience. Thomson Wadsworth.
Marie T. Banich (1997). Neuropsychology. The neural bases of Mental Function. Houghton Mifflin.
Wilson, Robert A.& Keil, Frank C. (1999). The MIT Encyclopedia of the Cognitive Sciences. Massachusetts: Bradford Book.
Ward, Jamie (2006). The Student’s Guide To Cognitive Science. Psychology Press. Levitin, D. J.(2002). Foundations of Cognitve Psychology.
Schmalhofer, Franz. Slides from the course: Cognitive Psychology and Neuropsychology, Summer Term 2006/2007, University of Osnabrueck
CHAPTER 11 LICENSE AND ATTRIBUTION
Source: Multiple authors. Memory. In Cognitive Psychology and Cognitive Neuroscience. Wikibooks. Retrieved from https://en.wikibooks.org/wiki/ Cognitive_Psychology_and_Cognitive_Neuroscience
Wikibooks are licensed under the Creative Commons Attribution-ShareAlike License.
Cognitive Psychology and Cognitive Neuroscience is licensed under the GNU Free Documentation License.
Condensed from original version. American spellings used. Content added or changed to reflect American perspective and references. Context and transitions added throughout. Substantially edited, adapted, and (in some parts) rewritten for clarity and course relevance.
Cover photo by Qurratul Ayin Sadia on Unsplash.